The future of AI in finance isn’t some far-off concept. It’s already showing up in real-world planning, forecasting, and decision-making. Teams are shifting from manual work and static reports to systems that update, learn, and adjust on their own.
Finance is changing, and it’s happening fast.
Gartner reports that 64% of CFOs expect to run autonomous finance models within six years. That puts the target at 2030. And companies like Siemens and OpenAI are already moving in that direction.
Read: Finance Automation in 2025: Tools, Use Cases, and Real-World Strategy
While some teams are experimenting with AI, many are still busy fixing broken spreadsheets or reconciling data manually. That gap will define the next five years. Not just in tools, but in how finance contributes to strategy.
How AI Is Already Changing the Way Finance Works
Finance teams aren’t waiting for the future. They’re already using AI to solve real problems, every day.
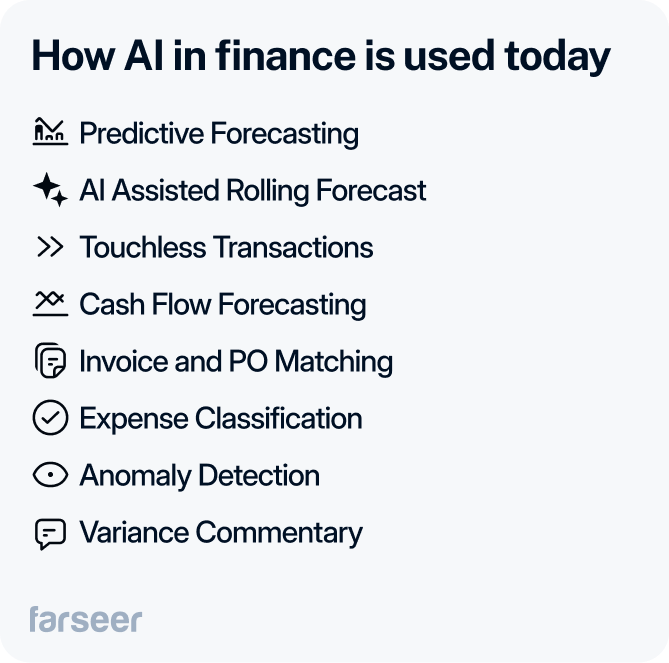
Predictive forecasting is one of the most common wins. AI models help spot shifts in revenue, cost, or cash flow before they show up in reports. At JPMorgan Chase, an AI-driven cash flow forecasting tool has reduced manual work for corporate clients by nearly 90%, helping teams gain better visibility and act faster.
Meanwhile, rolling forecasts now update automatically as new sales or market data comes in. AI models adjust assumptions in real-time based on trends in pipeline, inventory, or FX, so the plan stays current without a full rework. Teams use this to react faster and avoid the lag of traditional planning cycles.
On top of that, touchless transactions are already replacing manual work. AI handles reconciliations, invoice coding, and journal entries at scale. Deloitte calls this setup the “Finance Factory.” If you’re looking for tools that support this kind of automation, we broke down how leading enterprise finance platforms apply AI to planning and operations. See which ones actually deliver the best AI for finance in our guide.
In addition, cash flow forecasting is getting more detailed. Some teams use AI to predict daily liquidity, factoring in payment behavior and external data.
Similarly, invoice and PO matching is faster now. AI can match documents even when they aren’t perfect.
Moreover, expense classification is easier too. AI sorts costs and routes approvals based on policies it learns over time.
Furthermore, anomaly detection helps teams catch fraud and reporting errors earlier.
Finally, variance commentary is no longer a manual chore. AI can now draft explanations, so teams can spend more time on actual analysis.
If you want to see how this works in practice, check out our AI in accounting deep-dive. For broader planning workflows, here’s how Farseer simplifies budgeting and forecasting.
The Future of AI in Finance: From Smart Tools to Autonomous FP&A
Finance teams have automated a lot of the basics. The next step is making planning systems smart enough to react on their own, without waiting for someone to update a spreadsheet or re-run a model.
The future of AI in finance is about building systems that continuously learn, adapt, and support decision-making at every level. Autonomous FP&A is what that looks like. These systems learn from business activity, adjust assumptions on the fly, and update plans in real-time. Many finance leaders expect to get there by 2030, and some are already building toward it. If you’re heading in that direction, this FP&A software guide highlights what to focus on first.
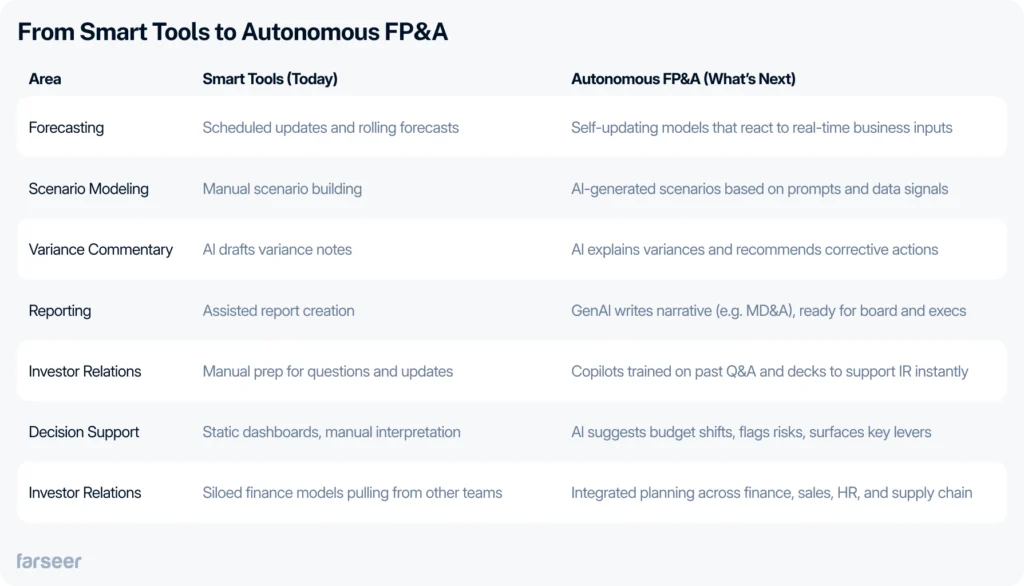
Generative AI will make this shift faster. Instead of building scenarios manually, you can ask AI to test what happens if prices drop, volume shifts, or a region underperforms. MIT’s Michael Schrage put it clearly: “GenAI will challenge the assumptions behind the numbers.”
Finance copilots are evolving fast. At OpenAI, the CFO’s team already uses a GPT-based assistant to answer investor questions and support close tasks. It’s not just pulling data, but explaining it. Tools like these can also draft MD&A (Management Discussion and Analysis) sections, answer variance questions, and build reports that are ready to share with stakeholders. As BCG puts it: “Finance copilots will explain results and recommend next steps.”
The next step is real decision support – recommending budget shifts, flagging cost risks, and highlighting the biggest levers based on live data.
And this won’t stay inside finance. Autonomous planning will connect with sales, HR, and supply chain, so the entire business can plan on shared, real-time inputs.
How to Get Started with AI in Finance, Without Breaking Things
AI won’t fix broken data or disconnected processes. It just makes the mess harder to spot.
The finance teams that get this right don’t chase flashy tools. They start small — with specific, logic-heavy workflows that drain time but follow clear rules. Think reconciliations, reforecasting, variance explanations. These aren’t experiments. They’re repeatable, data-driven tasks that give you fast returns when automated properly.
Before doing anything, check your systems. If actuals come from five sources or your cost center mapping is inconsistent, AI won’t solve it — it’ll just move faster through bad inputs. Clean data isn’t a phase; it’s a requirement.
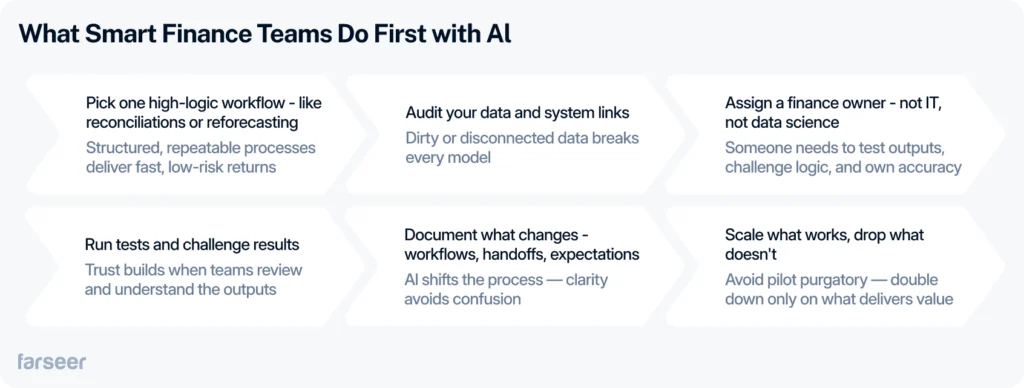
Then make sure someone owns the process. You don’t need an AI expert. You need a finance lead who knows how the model works, where the numbers come from, and when the output feels off. That’s the person who keeps everything grounded.
Once the tools are in place, the job changes. You’ll stop rebuilding plans from scratch and spend more time stress-testing them. You won’t write commentary line by line — you’ll review what AI drafts and focus on what needs context.
One thing most teams miss? The reaction. AI will start recommending changes. Not everyone will trust them right away. That’s normal. The best teams involve stakeholders early. They test outputs together. That’s what builds trust and makes the results stick.
Start with what’s slow and structured. Make it work. Then scale.
Future of AI in Finance Depends on Managing Risk
AI speeds things up, but if you don’t manage the risks, it also speeds up mistakes.
Bias creeps in fast. If your past budgets were skewed or built on outdated assumptions, AI will repeat them. It learns from what you feed it. The U.S. Treasury already flagged bias as a real risk in financial systems.
Black-box models don’t fly in finance. You still need to explain your forecast to auditors and the board. If no one on your team can say why the number changed, you shouldn’t use it. Stick to models you can trace and test. Keep a human in the loop.
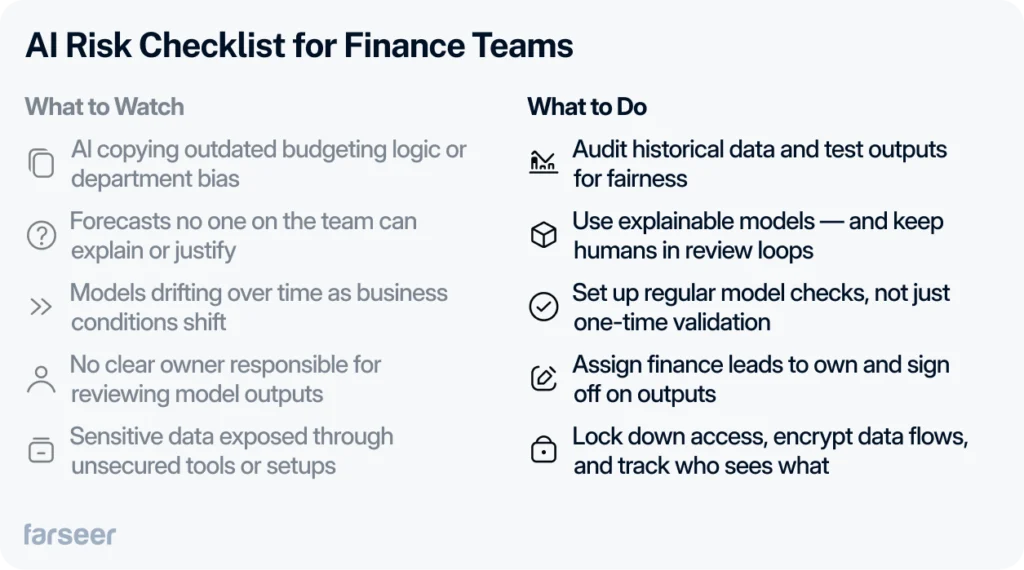
Someone has to own the output. That means assigning model owners — people who check the logic, track changes, and sign off on what goes to leadership. The CFO is still on the hook for decisions, even if AI helped shape them.
Security has to scale with the tech. AI tools handle sensitive data: forecasts, salary bands, M&A plans. Lock that down. Use encrypted pipelines, restrict access by role, and document who sees what.
And don’t assume the model will keep working as expected. Business shifts, inputs drift, logic breaks. Someone needs to keep testing and adjusting, or the results will quietly stop making sense.
What Comes Next
AI in finance won’t stop at speeding up tasks. These tools are already learning, adjusting, and turning data into insight — all in real time. The next step is autonomy: systems that support finance as a decision-maker, not just a reporter.
By 2030, planning won’t happen once a quarter. It’ll stay updated as conditions change. Forecasts won’t be rebuilt — they’ll be refined. And reporting won’t just explain the past — it’ll highlight what matters now, and what to do next.
The shift is already underway. Teams that start shaping it now will be the ones driving the business forward.